Do the Divergent Results of COVID-19 Antibody Studies Reflect Real Differences?
The infection fatality rate probably varies from one place to another.
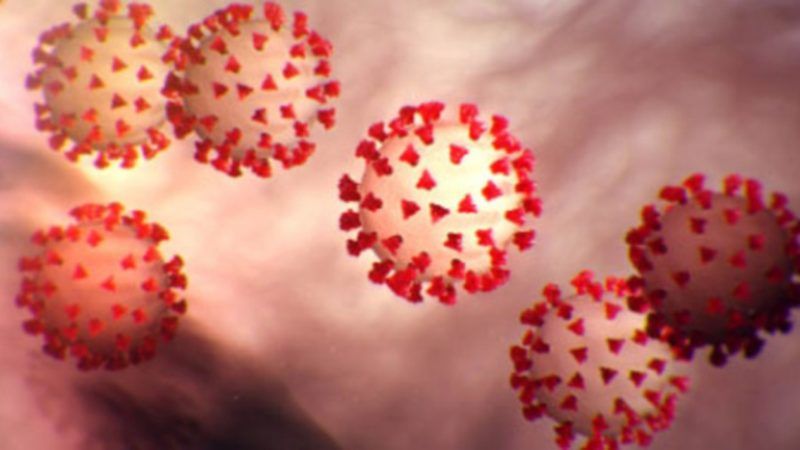
How deadly is COVID-19? It has become increasingly clear that the answer varies from place to place. Local conditions affect not only the percentage of the population that is infected but also the percentage of those people who die as a result.
That point has been largely overlooked in the often heated debate about U.S. antibody studies, which has focused on methodological issues such as sampling bias and the accuracy of the tests used to determine who has been infected by the COVID-19 virus. While those issues are important, it's misleading to assume that we are trying to identify the one true infection fatality rate (IFR) for the entire country, let alone the world.
Two Australian public health researchers, Gideon Meyerowitz-Katz and Lea Merone, recently reviewed 13 studies that used various methods, including virus tests, antibody tests, "excess deaths," and epidemiological modeling, to estimate the IFR for COVID-19. The estimates covered a wide range, from 0.05 percent in Iceland to 1.3 percent in Northern Italy and among the passengers and crew of the Diamond Princess cruise ship.
The weighted average was 0.75 percent. But "due to very high heterogeneity in the meta-analysis," Meyerowitz-Katz and Merone warn, "it is difficult to know if this represents the 'true' point estimate. It is likely that different places will experience different IFRs. More research looking at age-stratified IFR is urgently needed to inform policy-making on this front."
It is clear that the COVID-19 death rate varies with age. A study of excess deaths in several Italian towns, based on a comparison of mortality during the pandemic to mortality during the same period in earlier years, found that the IFR ranged from 0.02 percent among people in their 40s to more than 15 percent among people older than 90. Another Italian study found an overall IFR of 1.3 percent, rising to 4.3 percent among people older than 60.
Age demographics help explain why death rates in Italy (median age: 47.3) and among people infected on the cruise ship (mean age: 58) were relatively high. "One reason for the very high heterogeneity is likely that different countries will experience different death rates due to the disease," Meyerowitz-Katz and Merone note. "It is very likely, given the evidence around age-related fatality, that a country with a significantly younger population would see fewer deaths on average than one with a far older population, given similar levels of healthcare provision between the two. For example, Israel, with a median age of 30 years, would expect a lower IFR than Italy, with a much higher median age."
Alan Reynolds, a senior fellow at the Cato Institute, argues that age is a proxy for serious preexisting medical conditions, which are also associated with a much higher death rate and become more common as people get older. He notes that underlying conditions such as diabetes, heart disease, liver disease, kidney disease, respiratory illnesses, and immune system suppression have been involved in at least 99 percent of COVID-19 deaths in New York City.
"The absolutely critical and widely misunderstood point here is that 'underlying conditions' are THE only risk that virtually all fatal cases of COVID-19 had in common—not age," Reynolds writes. "That misunderstanding arose because old people are far more likely to have one or more of these conditions (and because more old people die of this and almost every other fatal risk). But it's about time to stop echoing the fallacy that this virus kills old people, rather than sick people."
The prevalence of preexisting health problems is obviously relevant in understanding why COVID-19 seems to be especially deadly in some places. Another widely cited explanation is the quality and capacity of the local health care system. Other things being equal, it makes sense that a jurisdiction where hospitals are stressed by a large number of COVID-19 cases would see not just more deaths but a higher IFR.
Antibody studies in New York and Indiana have yielded estimated IFRs of about 0.6 percent, three times the estimates from antibody studies in Miami-Dade County, Los Angeles County, and Santa Clara County. Some of that gap may be due to methodological problems with the latter studies, which could have exaggerated the prevalence of infection and therefore underestimated the IFR.
The Santa Clara County study, which was conducted by researchers at Stanford University, has been widely criticized. The authors respond to that criticism in the latest version of their preprint. One of the study's harshest critics, Columbia University statistician Andrew Gelman, offers a mixed review of the revised version.
One outstanding issue regarding the Santa Clara County study is its solicitation of subjects through Facebook ads, which may have biased the sample toward people who were especially eager to be tested because they were especially likely to have been infected. The researchers in Los Angeles County and Miami-Dade, by contrast, used random samples designed to be representative of the local population, as did the researchers in Indiana. The authors of the Los Angeles County study, which was published today as a letter to The Journal of the American Medical Association, nevertheless note that "the estimated prevalence may be biased" because "symptomatic persons may have been more likely to participate." The New York subjects were randomly selected from shoppers, which could have created a bias in either direction.
Another major issue is the accuracy of the antibody tests—in particular, their specificity, which indicates how often they correctly identified negative samples as negative in validation tests. Even a seemingly high specificity (say, 90 percent) can generate more false positives than true positives when researchers test subjects from a population in which the prevalence of infection is relatively low.
The New York study used a test developed by the state health department that has been validated by the Food and Drug Administration (FDA). According to the FDA, that kit has a specificity of 98.8 percent, meaning it incorrectly identified negative samples as positive about 1 percent of the time. Assuming that 5 percent of the population has been infected, the FDA says, the New York test has a positive predictive value of 79.4 percent, meaning roughly one in five positive results will be wrong. The Indiana study used Abbott's IgG test, which according to the FDA has a specificity of 99 percent and a positive predictive value of 84 percent when prevalence is 5 percent.
The Los Angeles County and Santa Clara County studies both used tests manufactured by the Chinese company Hangzhou Biotest Biotech and distributed in the United States by Premier Biotech, which is based in Minneapolis. The manufacturer reported a specificity of 99.5 percent, but that rate has not been validated by the FDA, and the Stanford researchers who conducted the Santa Clara study independently tested just a small number of validation samples. The actual specificity of the Premier Biotech kits, which is important in the prevalence calculation and therefore the IFR calculation, is a major point of contention between the Stanford researchers and critics of the study.
The Miami-Dade study used tests produced by the North Carolina company BioMedomics. The company reports that its test, which has not been validated by the FDA, generated 12 false positives out of 128 samples from uninfected people, meaning the results were erroneous more than 9 percent of the time. If the true prevalence of infection were 5 percent, the BioMedomics test would generate more false positives than true positives. It's not clear whether and to what extent the University of Miami researchers who conducted the study took false positives into account. That study, like the New York and Indiana studies, has not been published, even as a preprint.
In short, methodological weaknesses—in particular, low test specificity—could help explain why the California and Florida studies generated much lower IFR estimates than the New York and Indiana studies. But that is not the whole story.
The crude case fatality rate (CFR)—known deaths as share of reported cases—is substantially higher in New York (nearly 8 percent) and Indiana (6.2 percent) than in California (4 percent) and Florida (4.3 percent). For reasons that may include overburdened hospitals, COVID-19 patients seem to have fared worse in New York and Indiana than they have in California and Florida. In other words, the divergent results may to some extent reflect actual differences in fatality rates.
The authors of the Santa Clara County study note that the IFR may "be substantially higher in places where the hospitals are overwhelmed (e.g. New York City or Bergamo), or where infections are concentrated among vulnerable individuals (e.g. populations without access to healthcare or nursing home residents). For example, in many European countries, 42–57% of deaths occurred in nursing homes and
the same appears to be true for 25% of deaths in New York. Infection fatality rate estimates may be substantially higher in such settings." While this interpretation is obviously appealing as a response to the study's critics, that does not mean it is wrong.
There are similar variations in IFR estimates from Europe. Antibody tests in Gangelt, Germany, covering some 80 percent of the local population, found that 15 percent of residents had been infected, suggesting an IFR of 0.4 percent—half the estimate for France and less than one-third the estimate for Northern Italy in studies considered by Meyerowitz-Katz and Merone. The difference is not surprising, given that Germany's crude CFR (4.6 percent) is much lower than the crude CFRs for France (15.7 percent) and Italy (14.2 percent).
In Sweden, meanwhile, the Public Health Authority recently raised its IFR estimate to 0.6 percent, which is similar to the estimates from New York and Indiana but still substantially lower than the estimates from Italy and France. Not everyone agrees with the new estimate for Sweden. "State epidemiologist Anders Tegnell and Anders Wallensten have both said they believe the mortality rate is below 0.4 percent," Sveriges Radio reports.
The plausibility of relatively low IFR estimates ultimately depends on the plausibility of the prevalence estimates on which that calculation relies. The latest version of the Santa Clara County study, for example, estimates that nearly 3 percent of the local population had been infected by early April, about the same as the prevalence estimate for Indiana in the last week of that month. Since Indiana reported its first COVID-19 death on March 16, more than a month after the first confirmed death in Santa Clara County, the prevalence estimate for the latter jurisdiction seems, if anything, surprisingly low.
It is still possible, of course, that the Stanford researchers substantially overestimated the prevalence of infection in Santa Clara County, depending on the impact of sampling bias and the actual specificity of the antibody test they used. Yet even if their IFR estimate is too low, it seems likely that the true IFR in Santa Clara County (and in California generally) is lower than the estimated IFRs in New York and Indiana, given the relatively high death rates among known cases in both of those places.
In other words, the contrasting results of the antibody studies may reflect both methodological issues and differences in the underlying reality. That possibility tends to get lost in the argument between people who uncritically embrace low IFR estimates and people who automatically dismiss them.
[This post has been updated to note today's publication of the Los Angeles County antibody study.]
Show Comments (28)