Stephen Wolfram on the Powerful Unpredictability of AI
A physicist considers whether artificial intelligence can fix science, regulation, and innovation.
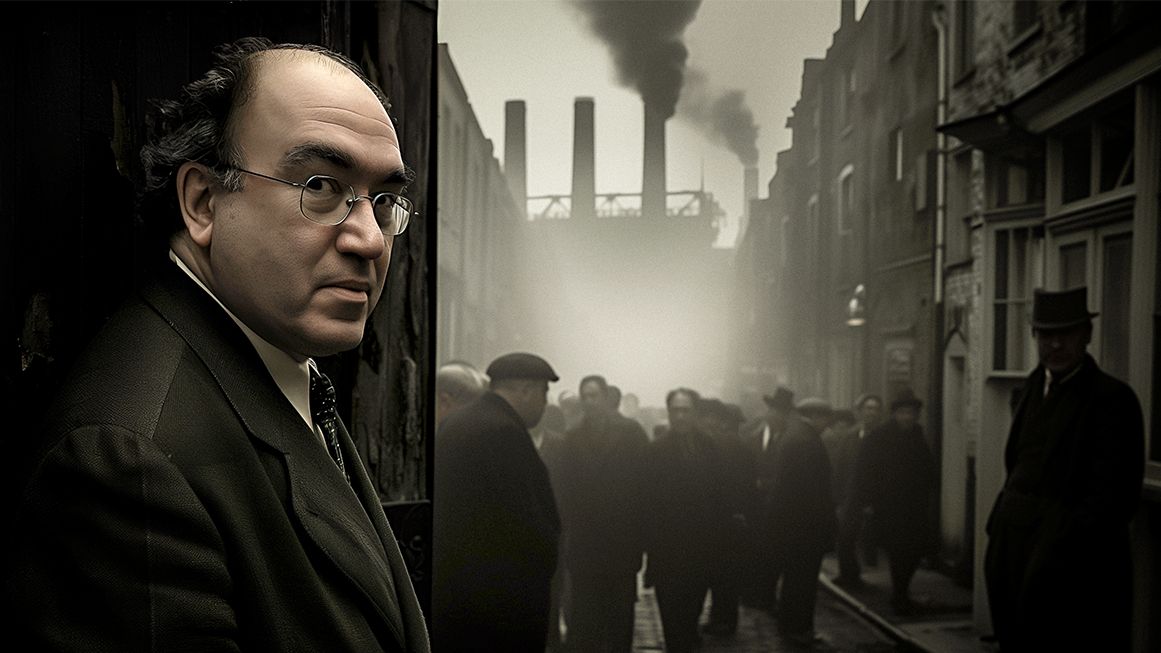
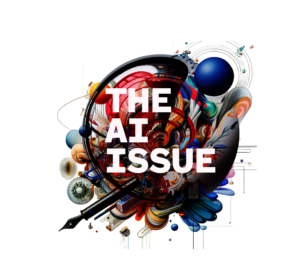
Stephen Wolfram is, strictly speaking, a high school and college dropout: He left both Eton and Oxford early, citing boredom. At 20, he received his doctorate in theoretical physics from Caltech and then joined the faculty in 1979. But he eventually moved away from academia, focusing instead on building a series of popular, powerful, and often eponymous research tools: Mathematica, WolframAlpha, and the Wolfram Language. He self-published a 1,200-page work called A New Kind of Science arguing that nature runs on ultrasimple computational rules. The book enjoyed surprising popular acclaim.
Wolfram's work on computational thinking forms the basis of intelligent assistants, such as Siri. In an April conversation with Reason's Katherine Mangu-Ward, he offered a candid assessment of what he hopes and fears from artificial intelligence, and the complicated relationship between humans and their technology.
Reason: Are we too panicked about the rise of AI or are we not panicked enough?
Wolfram: Depends who "we" is. I interact with lots of people and it ranges from people who are convinced that AIs are going to eat us all to people who say AIs are really stupid and won't be able to do anything interesting. It's a pretty broad range.
Throughout human history, the one thing that's progressively changed is the development of technology. And technology is often about automating things that we used to have to do ourselves. I think the great thing technology has done is provide this taller and taller platform of what becomes possible for us to do. And I think the AI moment that we're in right now is one where that platform just got ratcheted up a bit.
You recently wrote an essay asking, "Can AI Solve Science?" What does it mean to solve science?
One of the things that we've come to expect is, science will predict what will happen. So can AI jump ahead and figure out what will happen, or are we stuck with this irreducible computation that has to be done where we can't expect to jump ahead and predict what will happen?
AI, as currently conceived, typically means neural networks that have been trained from data about what humans do. Then the idea is, take those training examples and extrapolate from those in a way that is similar to the way that humans would extrapolate.
Now can you turn that on science and say, "Predict what's going to happen next, just like you can predict what the next word should be in a piece of text"? And the answer is, well, no, not really.
One of the things we've learned from the large language models [LLMs] is that language is easier to predict than we thought. Scientific problems run right into this phenomenon I call computational irreducibility—to know what's going to happen, you have to explicitly run the rules.
Language is something we humans have created and use. Something about the physical world just delivered that to us. It's not something that we humans invented. And it turns out that neural nets work well on things that we humans invented. They don't work very well on things that are just sort of wheeled in from the outside world.
Probably the reason that they work well on things that we humans invented is that their actual structure and operation is similar to the structure and operation of our brains. It's asking a brainlike thing to do brainlike things. So yes, it works, but there's no guarantee that brainlike things can understand the natural world.
That sounds very simple, very straightforward. And that explanation is not going to stop entire disciplines from throwing themselves at that wall for a little while. This feels like it's going to make the crisis in scientific research worse before it gets better. Is that too pessimistic?
It used to be the case that if you saw a big, long document, you knew that effort had to be put into producing it. That suddenly became not the case. They could have just pressed a button and got a machine to generate those words.
So now what does it mean to do a valid piece of academic work? My own view is that what can be most built upon is something that is formalized.
For example, mathematics provides a formalized area where you describe something in precise definitions. It becomes a brick that people can expect to build on.
If you write an academic paper, it's just a bunch of words. Who knows whether there's a brick there that people can build on?
In the past we've had no way to look at some student working through a problem and say, "Hey, here's where you went wrong," except for a human doing that. The LLMs seem to be able to do some of that. That's an interesting inversion of the problem. Yes, you can generate these things with an LLM, but you can also have an LLM understand what was happening.
We are actually trying to build an AI tutor—a system that can do personalized tutoring using LLM. It's a hard problem. The first things you try work for the two-minute demo and then fall over horribly. It's actually quite difficult.
What becomes possible is you can have the [LLM] couch every math problem in terms of the particular thing you are interested in—cooking or gardening or baseball—which is nice. It's a sort of a new level of human interface.
So I think that's a positive piece of what becomes possible. But the key thing to understand is the idea that an essay means somebody committed to write an essay is no longer a thing.
We're going to have to let that go.
Right. I think the thing to realize about AIs for language is that what they provide is kind of a linguistic user interface. A typical use case might be you are trying to write some report for some regulatory filing. You've got five points you want to make, but you need to file a document.
So you make those five points. You feed it to the LLM. The LLM puffs out this whole document. You send it in. The agency that's reading it has their own LLM, and they're asking their LLM, "Find out the two things we want to know from this big regulatory filing." And it condenses it down to that.
So essentially what's happened is you've used natural language as a sort of transport layer that allows you to interface one system to another.
I have this deeply libertarian desire to say, "Could we skip the elaborate regulatory filing, and they could just tell the five things directly to the regulators?"
Well, also it's just convenient that you've got these two systems that are very different trying to talk to each other. Making those things match up is difficult, but if you have this layer of fluffy stuff in the middle, that is our natural language, it's actually easier to get these systems to talk to each other.
I've been pointing out that maybe 400 years ago was sort of a heyday of political philosophy and people inventing ideas about democracy and all those kinds of things. And I think that now there is a need and an opportunity for a repeat of that kind of thinking, because the world has changed.
As we think about AIs that end up having responsibilities in the world, how do we deal with that? I think it's an interesting moment when there should be a bunch of thinking going on about this. There is much less thinking than I think there should be.
An interesting thought experiment is what you might call the promptocracy model of government. One approach is everybody writes a little essay about how they want the world to be, and you feed all those essays into an AI. Then every time you want to make a decision, you just ask the AI based on all these essays that you read from all these people, "What should we do?"
One thing to realize is that in a sense, the operation of government is an attempt to make something like a machine. And in a sense, you put an AI in place rather than the human-operated machine, not sure how different it actually is, but you have these other possibilities.
The robot tutor and the government machine sound like stuff from the Isaac Asimov stories of my youth. That sounds both tempting and so dangerous when you think about how people have a way of bringing their baggage into their technology. Is there a way for us to work around that?
The point to realize is the technology itself has nothing. What we're doing with AI is kind of an amplified version of what we humans have.
The thing to realize is that the raw computational system can do many, many things, most of which we humans do not care about. So as we try and corral it to do things that we care about, we necessarily are pulling it in human directions.
What do you see as the role of competition in resolving some of these concerns? Does the intra-AI competition out there curb any ethical concerns, perhaps in the way that competition in a market might constrain behavior in some ways?
Interesting question. I do think that the society of AIs is more stable than the one AI that rules them all. At a superficial level it prevents certain kinds of totally crazy things from happening, but the reason that there are many LLMs is because once you know ChatGPT is possible, then it becomes not that difficult at some level. You see a lot of both companies and countries stepping up to say, "We'll spend the money. We'll build a thing like this." It's interesting what the improvement curve is going to look like from here. My own guess is that it goes in steps.
How are we going to screw this up? And by "we," I mean maybe people with power, maybe just general human tendencies, and by "this," I mean making productive use of AI.
The first thing to realize is AIs will be suggesting all kinds of things that one might do just as a GPS gives one directions for what one might do. And many people will just follow those suggestions. But one of the features it has is you can't predict everything about what it will do. And sometimes it will do things that aren't things we thought we wanted.
The alternative is to tie it down to the point where it will only do the things we want it to do and it will only do things we can predict it will do. And that will mean it can't do very much.
We arguably do the same thing with human beings already, right? We have lots of rules about what we don't let people do, and sometimes we probably suppress possible innovation on the part of those people.
Yes, that's true. It happens in science. It's a "be careful what you wish for" situation because you say, "I want lots of people to be doing this kind of science because it's really cool and things can be discovered." But as soon as lots of people are doing it, it ends up getting this institutional structure that makes it hard for new things to happen.
Is there a way to short circuit that? Or should we even want to?
I don't know. I've thought about this for basic science for a long time. Individual people can come up with original ideas. By the time it's institutionalized, that's much harder. Having said that: As the infrastructure of the world, which involves huge numbers of people, builds up, you suddenly get to this point where you can see some new creative thing to do, and you couldn't get there if it was just one person beavering away for decades. You need that collective effort to raise the whole platform.
This interview has been condensed and edited for style and clarity.
This article originally appeared in print under the headline "The Powerful Unpredictability of AI."
Show Comments (15)